14 Oct 2015
I’ve released a number of libraries for doing Markov Chain Monte Carlo (MCMC)
in Haskell.
You can get at them via a ‘frontend’ library,
declarative, but each can
also be used fruitfully on its own. À la carte, if you will.
Some background: MCMC is a family of stateful algorithms for sampling from a
large class of probability distributions. Typically one is interested in doing
this to approximate difficult integrals; instead of choosing some suitable grid
of points in parameter space over which to approximate an integral, just
offload the problem to probability theory and use a Markov chain to find them
for you.
For an excellent introduction to MCMC you won’t find better than Iain Murray’s
lectures from MLSS ’09 in
Cambridge, so check those out if you’re interested in more details.
I’ve put together a handful of popular MCMC algorithms as well as an easy way
to glue them together in a couple of useful ways. At present these
implementations are useful in cases where you can write your target function in
closed form, and that’s pretty much all that’s required (aside from the
standard algorithm-specific tuning parameters).
The API should be pretty easy to work with — write your target as a function of
its parameters, specify a start location, and away you go. It’s also cool if
your target accepts its parameters via most common traversable
functors — lists, vectors, sequences, maps, etc.
That’s sort of the goal of this first release: if you can give me a target
function, I’ll do my best to give you samples from it. Less is more and all
that.
What‘s In The Box
There are a number of libraries involved. I have a few more in the queue and
there are a number of additional features I plan to support for these ones in
particular, but without further ado:
- mwc-probability, a
sampling-function based probability monad implemented as a thin wrapper over
the excellent mwc-random
library.
- mcmc-types, housing a
number of types used by the the whole family.
- mighty-metropolis,
an implementation of the famous Metropolis algorithm.
- speedy-slice, a slice
sampling implementation suitable for both continuous & discrete parameter
spaces.
- hasty-hamiltonian,
an implementation of the gradient-based Hamiltonian Monte Carlo algorithm.
- declarative, the one ring
to rule them all.
Pull down declarative if you just want to have access to all of them. If
you’re a Haskell neophyte you can find installation instructions at the Github
repo.
Motivation
MCMC is fundamentally about observing Markov chains over probability spaces.
In this context a chain is a stochastic process that wanders around a state
space, eventually visiting regions of the space in proportion to their
probability.
Markov chains are constructed by transition operators that obey the Markov
property: that the probability of transitioning to the next
location — conditional on the history of the chain — depends only on the
current location. For MCMC we’re also interested in operators that satisfy the
reversibility property — that the probability a transition from state A to
state B occurs is the same as that a transition from state B to state A occurs.
A chain is characterized by a transition operator T that drives it from state
to state, and for MCMC we want the stationary or limiting distribution of the
chain to be the distribution we’re sampling from.
One of the major cottage industries in Bayesian research is inventing new
transition operators to drive the Markov chains used in MCMC. This has been
fruitful, but it could likely be aided by a practical way to make existing
transition operators work together.
This is easy to do in theory: there are a couple of ways to combine transition
operators such that the resulting composite operator preserves all the
properties we’re interested in for MCMC — the stationary distribution,
reversibility, and Markov property. See Geyer,
2005 for details here, but
the crux is that we can establish the following simple grammar for transition
operators:
transition ::= primitive <transition>
| concat transition transition
| sample transition transition
A transition is either some primitive operator, a deterministic concatenation
of operators (via ‘concat’), or a probabilistic concatenation of operators (via
‘sample’). A deterministic concatenation works by just transitioning through
two operators one after the other; a probabilistic concatenation works by
randomly choosing one transition operator or the other to use on any given
transition. These kinds of concatenation preserve all the properties we’re
interested in.
We can trivially generalize this further by adding a term that concatenates n
transition operators together deterministically, or another for
probabilistically concatenating a bunch of operators according to some desired
probability distribution.
The idea here is that there are tradeoffs involved in different transition
operators. Some may be more computationally expensive than others (perhaps
requiring a gradient evaluation, or evaluation of some inner loop) but have
better ability to make ‘good’ transitions in certain situations. Other
operators are cheap, but can be inefficient (taking a long time to visit
certain regions of the space).
By employing deterministic or probabilistic concatenation, one can concoct a
Markov chain that uses a varied range of tuning parameters, for example. Or
only occasionally employs a computationally expensive transition, otherwise
preferring some cheaper, reliable operator.
Usage
The declarative library implements this simple language for transition
operators, and the mighty-metropolis, speedy-slice, and hasty-hamiltonian
libraries provide some primitive transitions that you can combine as needed.
The Metropolis and slice sampling transitions are cheap and require little
information, whereas Hamiltonian Monte Carlo exploits information about the
target’s gradient and also involves evaluation of an inner loop (the length of
which is determined by a tuning parameter). Feel free to use one that suits
your problem, or combine them together using the combinators supplied in
declarative to build a custom solution.
As an example, the Rosenbrock
density is a great test
dummy as it’s simple, low-dimensional, and can be easily visualized, but it
still exhibits a pathological anisotropic structure that makes it somewhat
tricky to sample from.
Getting started via declarative is pretty simple:
You’ll want to supply a target to sample over, and if you want to use an
algorithm like Hamiltonian Monte Carlo you’ll also need to provide a gradient.
If you can’t be bothered to calculate gradients by hand, you can always turn to
your friend automatic
differentiation:
The Rosenbrock log-density and its gradient can then be written as follows:
target :: Num a => [a] -> a
target [x0, x1] = negate (100 * (x1 — x0 ^ 2) ^ 2 + (1 — x0) ^ 2)
gTarget :: Num a => [a] -> [a]
gTarget = grad target
All you need to do here is provide a function proportional to a
log-probability density. The logarithmic scale is important; various internals
expect to be passed (something proportional to) a log-probability density.
To package these guys up together we can wrap them in a Target
. Note that we
don’t always care about including a gradient, so that part is optional:
rosenbrock :: Target [Double]
rosenbrock = Target target (Just gTarget)
The Target
type is parameterized over the shape of the parameter space. You
could similarly have a Target (Seq Double)
, Target (Map String Double)
, and
so on. Your target may be implemented using a boxed vector for efficiency, for
example. Or using a Map or HashMap with string/text keys such that parameter
names are preserved. They should work just fine.
Given a target, we can sample from it a bunch of times using a simple
Metropolis transition via the mcmc function. Aside from the target and a
PRNG, provide it with the desired number of transitions, a starting point, and
the transition operator to use:
> -- haskell
> prng <- create
> mcmc 10000 [0, 0] (metropolis 1) rosenbrock prng
In return you’ll get the desired trace of the chain dumped to stdout:
8.136972300105949e-2,0.273896953404261
0.4657348148676972,0.17462596647788464
-0.48609414127836326,9.465052854751566e-2
-0.49781488399832785,0.42092910345708523
-0.3019713424699155,0.39135350029173566
0.12058426470979189,0.12485407390388925
..
The intent is for the chain to be processed elsewhere — if you’re me, that will
usually be in R. Libraries like
coda have a ton of
functionality useful for working with Markov chain traces, and
ggplot2 as a library for static statistical graphics
can’t really be beat:
> # r
> d = read.csv(‘rosenbrock-trace.dat’, header = F)
> names(d) = c(‘x’, ‘y’)
> require(ggplot2)
> ggplot(d, aes(x, y)) + geom_point(colour = ‘darkblue’, alpha = 0.2)
You get the following trace over the Rosenbrock density, taken for 10k
iterations. This is using a Metropolis transition with variance 1:
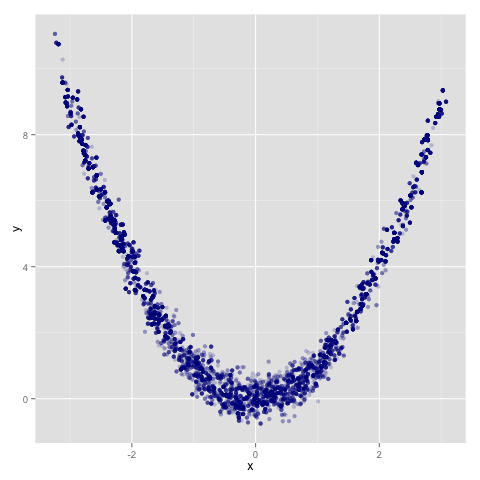
If you do want to work with chains in memory in Haskell you can do that by
writing your own handling code around the supplied transition operators. I’ll
probably make this a little easier in later versions.
The implementations are reasonably quick and don’t leak memory — the traces are
streamed to stdout as the chains are traversed. Compiling the above with ‘-O2’
and running it for 100k iterations yields the following performance
characteristics on my mid-2011 model MacBook Air:
$ ./test/Rosenbrock +RTS -s > /dev/null
3,837,201,632 bytes allocated in the heap
8,453,696 bytes copied during GC
89,600 bytes maximum residency (2 sample(s))
23,288 bytes maximum slop
1 MB total memory in use (0 MB lost due to fragmentation)
INIT time 0.000s ( 0.000s elapsed)
MUT time 3.539s ( 3.598s elapsed)
GC time 0.049s ( 0.058s elapsed)
EXIT time 0.000s ( 0.000s elapsed)
Total time 3.591s ( 3.656s elapsed)
%GC time 1.4% (1.6% elapsed)
Alloc rate 1,084,200,280 bytes per MUT second
Productivity 98.6% of total user, 96.8% of total elapsed
The beauty is that rather than running a chain solely on something like the
simple Metropolis operator used above, you can sort of ‘hedge your sampling
risk’ and use a composite operator that proposes transitions using a multitude
of ways. Consider this guy, for example:
transition =
concatT
(sampleT (metropolis 0.5) (metropolis 1.0))
(sampleT (slice 2.0) (slice 3.0))
Here concatT
and sampleT
correspond to the concat
and sample
terms in
the BNF description in the previous section. This operator performs two
transitions back-to-back; the first is randomly a Metropolis transition with
standard deviation 0.5 or 1 respectively, and the second is a slice sampling
transition using a step size of 2 or 3, randomly.
Running it for 5000 iterations (to keep the total computation approximately
constant), we see a chain that has traversed the space a little better:
> mcmc 5000 [0, 0] transition rosenbrock prng
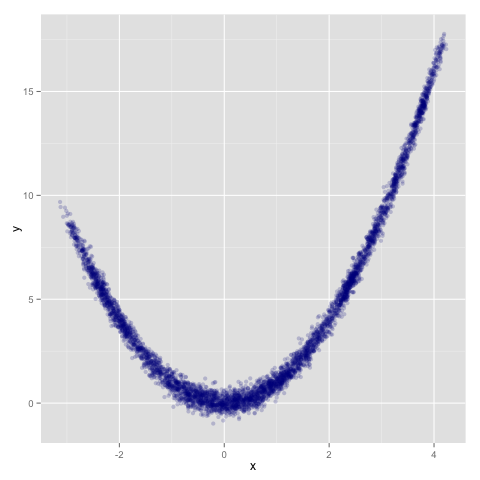
It’s worth noting that I didn’t put any work into coming up with this composite
transition: this was just the first example I thought up, and a lot of the
benefits here probably come primarily from including the eminently-reliable
slice sampling transition. But from informal experimentation, it does seem that
chains driven by composite transitions involving numerous operators and tuning
parameter settings often seem to perform better on average than a given chain
driven by a single (poorly-selected) transition.
I know exactly how meticulous proofs and benchmarks must be so I haven’t
rigorously established any properties around this, but hey: it ‘seems to be the
case’, and intuitively, including varied transition operators surely hedges
your bets when compared to using a single one.
Try it out and see how your mileage varies, and be sure to let me know if you
find some killer apps where composite transitions really seem to win.
Implementation Notes
If you’re just interested in using the libraries you can skip the following
section, but I just want to point out how easy this is to implement.
The implementations are defined using a small set of types living in
mcmc-types:
type Transition m a = StateT a (Prob m) ()
data Chain a b = Chain {
chainTarget :: Target a
, chainScore :: Double
, chainPosition :: a
, chainTunables :: Maybe b
}
data Target a = Target {
lTarget :: a -> Double
, glTarget :: Maybe (a -> a)
}
Most important here is the Transition
type, which is just a state transformer
over a probability monad (itself defined in mwc-probability). The probability
monad is the source of randomness used to define transition operators useful
for MCMC, and values with type Transition
are the transition operators in
question.
The Chain
type is the state of the Markov chain at any given iteration. All
that’s really required here is the chainPosition
field, which represents the
location of the chain in parameter space. But adding some additional
information here is convenient; chainScore
caches the most recent score of
the chain (which is typically used in internal calculations, and caching avoids
recomputing things needlessly) and chainTunables
is an optional record
intended to be used for stateful tuning parameters (used by adaptive algorithms
or in burn-in phases and the like). Additionally the target being sampled from
itself — chainTarget
— is included in the state.
Undisciplined use of chainTarget
and chainTunables
can have all sorts of
nasty consequences — you can use them to change the stationary distribution
you’re sampling from or invalidate the Markov property — but keeping them
around is useful for implementing some desirable features. Tweaking
chainTarget
, for example, allows one to easily implement annealing, which can
be very useful for sampling from annoying multi-modal densities.
Setting everything up like this makes it trivial to mix-and-match transition
operators as required — the state and probability monad stack provides
everything we need. Deterministic concatenation is implemented as follows, for
example:
and a generalized version of probabilistic concatenation just requires a coin
flip:
bernoulliT p t0 t1 = do
heads <- lift (MWC.bernoulli p)
if heads then t0 else t1
A uniform probabilistic concatenation over two operators, implemented in
sampleT
, is then just bernoulliT 0.5
.
The difficulty of implementing primitive operators just depends on the operator
itself; the surrounding framework is extremely lightweight. Here’s the
Metropolis transition, for example (with type signatures omitted to keep the
noise down):
metropolis radial = do
Chain {..} <- get
proposal <- lift (propose radial chainPosition)
let proposalScore = lTarget chainTarget proposal
acceptProb = whenNaN 0
(exp (min 0 (proposalScore - chainScore)))
accept <- lift (MWC.bernoulli acceptProb)
when accept
(put (Chain chainTarget proposalScore proposal chainTunables))
propose radial = traverse perturb where
perturb m = MWC.normal m radial
And the excellent pipes library is
used to generate a Markov chain:
chain radial = loop where
loop state prng = do
next <- lift
(MWC.sample (execStateT (metropolis radial) state) prng)
yield next
loop next prng
The mcmc
functions are also implemented using pipes. Take the first n
iterations of a chain and print them to stdout. That simple.
Future Work
In the near term I plan on updating some old MCMC implementations I have
kicking around on Github (flat-mcmc,
lazy-langevin,
hnuts) and releasing them within this
framework. Additionally I’ve got some code for building annealed operators that
I want to release — it has been useful in some situations when sampling from
things like the Himmelblau
density, which has a
few disparate clumps of probability that make it tricky to sample from with
conventional algorithms.
This framework is also useful as an inference backend to languages for working
with directed graphical models (think BUGS/Stan). The idea here is that you
don’t need to specify your target function (typically a posterior density)
explicitly: just describe your model and I’ll give you samples from the
posterior distribution. A similar version has been put to use around the
BayesHive project.
Longer term — I’ll have to see what’s up in terms of demand. There are
performance improvements and straightforward extensions to things like
parallel tempering, but I’m
growing more interested in ‘online’ methods like particle
MCMC
and friends that are proving useful for inference in more general probabilistic
programs (think those expressible by Church and its
ilk).
Let me know if you get any use out of these things, or please file an issue if
there’s some particular feature you’d like to see supported.
Thanks to Niffe Hermansson for review and helpful comments.
06 Sep 2015
Recursion schemes are elegant and useful patterns for expressing general
computation. In particular, they allow you to ‘factor recursion out’ of
whatever semantics you may be trying to express when interpreting programs,
keeping your interpreters concise, your concerns separated, and your code more
maintainable.
What’s more, formulating programs in terms of recursion schemes seems to help
suss out particular similarities in structure between what might be seen as
disparate problems in
other domains. So aside from being a practical computational tool, they seem to
be of some use when it comes to ‘hacking understanding’ in varied areas.
Unfortunately, they come with a pretty forbidding barrier to entry. While there
are a
few
nice
resources
out there for learning about recursion schemes and how they work, most
literature around them is quite
academic and
awash in some astoundingly technical jargon (more on this later). Fortunately,
the accessible resources out there do a great job of explaining what recursion
schemes are and how you might use them, so they go through some effort to build
up the required machinery from scratch.
In this article I want to avoid building up the machinery meticulously and
instead concentrate mostly on understanding and using Edward Kmett’s
recursion-schemes
library, which, while lacking in documentation, is very well put together and
implements all the background plumbing one needs to get started.
In particular, to feel comfortable using recursion-schemes I found that there
were a few key patterns worth understanding:
- Factoring recursion out of your data types using pattern functors and a
fixed-point wrapper.
- Using the ‘Foldable’ & ‘Unfoldable’ classes, plus navigating the ‘Base’ type
family.
- How to use some of the more common recursion schemes out there for everyday
tasks.
The Basics
If you’re following along in GHCi, I’m going to first bring in some
imports and add a useful pragma. I’ll dump a gist at the bottom; note that this
article targets GHC 7.10.2 and recursion-schemes-4.1.2, plus I’ll also require
data-ordlist-0.4.7.0 for an example later. Here’s the requisite boilerplate:
{-# LANGUAGE DeriveFunctor #-}
import Data.Functor.Foldable
import Data.List.Ordered (merge)
import Prelude hiding (Foldable, succ)
So, let’s get started.
Recursion schemes are applicable to data types that have a suitable recursive
structure. Lists, trees, and natural numbers are illustrative candidates.
Being so dead-simple, let’s take the natural numbers as an illustrative/toy
example. We can define them recursively as follows:
data Natural =
Zero
| Succ Natural
This is a fine definition, but many such recursive structures can also be
defined in a different way: we can first ‘factor out’ the recursion by defining
some base structure, and then ‘add it back in’ by using a recursive wrapper
type.
The price of this abstraction is a slightly more involved type definition, but
it unlocks some nice benefits — namely, the ability to reason about recursion
and base structures separate from each other. This turns out to be a very
useful pattern for getting up and running with recursion schemes.
The trick is to create a different, parameterized type, in which the new
parameter takes the place of all recursive points in the original type. We can
create this kind of base structure for the natural numbers example as follows:
data NatF r =
ZeroF
| SuccF r
deriving (Show, Functor)
This type must be a functor in this new parameter, so the type is often called
a ‘pattern functor’ for some other type. I like to use the notation
‘F’ when defining constructors for pattern functors.
We can define pattern functors for lists and trees in the same way:
data ListF a r =
NilF
| ConsF a r
deriving (Show, Functor)
data TreeF a r =
EmptyF
| LeafF a
| NodeF r r
deriving (Show, Functor)
Now, to add recursion to these pattern functors we’re going to use the famous
fixed-point type, ‘Fix’, to wrap them in:
type Nat = Fix NatF
type List a = Fix (ListF a)
type Tree a = Fix (TreeF a)
‘Fix’ is a standard fixed-point type imported from the recursion-schemes
library. You can get a ton of mileage from it. It introduces the ‘Fix’
constructor everywhere, but that’s actually not much of an issue in practice.
One thing I typically like to do is add some smart constructors to get around
it:
zero :: Nat
zero = Fix ZeroF
succ :: Nat -> Nat
succ = Fix . SuccF
nil :: List a
nil = Fix NilF
cons :: a -> List a -> List a
cons x xs = Fix (ConsF x xs)
Then you can write expressions like ‘succ (succ (succ zero))’ without having to
deal with the ‘Fix’ constructor explicitly. Note also that these expressions
are Showable à la carte, for example in GHCi:
> succ (succ (succ zero))
Fix (SuccF (Fix (SuccF (Fix (SuccF (Fix ZeroF))))))
A Short Digression on ‘Fix’
The ‘Fix’ type is brought into scope from ‘Data.Functor.Foldable’, but it’s
worth looking at it in some detail. It can be defined as follows, along with
two helpful functions for working with it:
newtype Fix f = Fix (f (Fix f))
fix :: f (Fix f) -> Fix f
fix = Fix
unfix :: Fix f -> f (Fix f)
unfix (Fix f) = f
‘Fix’ has a simple recursive structure. For a given value, you can think of
‘fix’ as adding one level of recursion to it. ‘unfix’ in turn removes one level
of recursion.
This generic recursive structure is what makes ‘Fix’ so useful: we can write
some nominally recursive type we’re interested in without actually using
recursion, but then package it up in ‘Fix’ to hijack the recursion it provides
automatically.
Understanding Some Internal Plumbing
If we wrap a pattern functor in ‘Fix’ then the underlying machinery of
recursion-schemes should ‘just work’. Here it’s worth explaining a little as to
why that’s the case.
There are two fundamental type classes involved in recursion-schemes:
‘Foldable’ and ‘Unfoldable’. These serve to tease apart the recursive structure
of something like ‘Fix’ even more: loosely, ‘Foldable’ corresponds to types
that can be ‘unfixed’, and ‘Unfoldable’ corresponds to types that can be
‘fixed’. That is, we can add more layers of recursion to instances of
‘Unfoldable’, and we can peel off layers of recursion from instances of
‘Foldable’.
In particular ‘Foldable’ and ‘Unfoldable’ contain functions called ‘project’
and ‘embed’ respectively, corresponding to more general forms of ‘unfix’ and
‘fix’. Their types are as follows:
project :: Foldable t => t -> Base t t
embed :: Unfoldable t => Base t t -> t
I’ve found it useful while using recursion-schemes to have a decent
understanding of how to interpret the type family ‘Base’. It appears frequently
in type signatures of various recursion schemes and being able to reason about
it can help a lot.
‘Base’ and Basic Type Families
Type families are type-level functions; they take types as input and return
types as output. The ‘Base’ definition in recursion-schemes looks like this:
type family Base t :: * -> *
You can interpret this as a function that takes one type ‘t’ as input and
returns some other type. An implementation of this function is called an
instance of the family. The instance for ‘Fix’, for example, looks like:
type instance Base (Fix f) = f
In particular, a type family like ‘Base’ is a synonym for instances of the
family. So using the above example: anywhere you see something like ‘Base (Fix
f)’ you can mentally replace it with ‘f’.
Instances of the ‘Base’ type family have a structure like ‘Fix’, but using
‘Base’ enables all the internal machinery of recursion-schemes to work
out-of-the-box for types other than ‘Fix’ alone. This has a typical Kmettian
flavour: first solve the most general problem, and then recover useful,
specific solutions to it automatically.
Predictably, ‘Fix f’ is an instance of ‘Base’, ‘Foldable’, and ‘Unfoldable’ for
some functor ‘f’, so if you use it, you can freely use all of
recursion-schemes’s innards without needing to manually write any instances for
your own data types. But as mentioned above, it’s worth noting that you can
exploit the various typeclass & type family machinery to get by without using
‘Fix’ at all: see e.g. Danny Gratzer’s recursion-schemes post for an example of
this.
Some Useful Schemes
So, with some discussion of the internals out of the way, we can look at some
of the more common and useful recursion schemes. I’ll concentrate on the
following four, as they’re the ones I’ve found the most use for:
- catamorphisms, implemented via ‘cata’, are generalized folds.
- anamorphisms, implemented via ‘ana’, are generalized unfolds.
- hylomorphisms, implemented via ‘hylo’, are anamorphisms followed by catamorphisms (corecursive production followed by recursive consumption).
- paramorphisms, implemented via ‘para’, are generalized folds with access to the input argument corresponding to the most recent state of the computation.
Let me digress slightly on nomenclature.
Yes, the names of these things are celebrations of the ridiculous. There’s no
getting around it; they look like self-parody to almost anyone not
pre-acquainted with categorical concepts. They have been accused — probably
correctly — of being off-putting.
That said, they communicate important technical details and are actually not so
bad when you get used to them. It’s perfectly fine and even encouraged to
arm-wave about folds or unfolds when speaking informally, but the moment
someone distinguishes one particular style of fold from another via a prefix
like e.g. para, I know exactly the relevant technical distinctions required to
understand the discussion. The names might be silly, but they have their place.
Anyway.
There are many other more exotic schemes that I’m sure are quite useful (see
Tim Williams’s recursion schemes talk, for example), but I haven’t made use of
any outside of these four just yet. The recursion-schemes library contains a
plethora of unfamiliar schemes just waiting to be grokked, but in the interim
even cata and ana alone will get you plenty far.
Now let’s use the motley crew of schemes to do some useful computation on our
example data types.
Catamorphisms
Take our natural numbers type, ‘Nat’. To start, we can use a catamorphism to
represent a ‘Nat’ as an ‘Int’ by summing it up.
natsum :: Nat -> Int
natsum = cata alg where
alg ZeroF = 0
alg (SuccF n) = n + 1
Here ‘alg’ refers to ‘algebra’, which is the function that we use to define our
reducing semantics. Notice that the semantics are not defined recursively! The
recursion present in ‘Nat’ has been decoupled and is handled for us by ‘cata’.
And as a plus, we still don’t have to deal with the ‘Fix’ constructor anywhere.
As a brief aside: I like to write my recursion schemes in this way, but your
mileage may vary. If you’d like to enable the ‘LambdaCase’ extension, then
another option is to elide mentioning the algebra altogether using a very
simple case statement:
{-# LANGUAGE LambdaCase #-}
natsum :: Nat -> Int
natsum = cata $ \case ->
ZeroF -> 0
SuccF n -> n + 1
Some people find this more readable.
To understand how we used ‘cata’ to build this function, take a look at its
type:
cata :: Foldable t => (Base t a -> a) -> t -> a
The ‘Base t a -> a’ term is the algebra; ‘t’ is our recursive datatype (i.e.
‘Nat’), and ‘a’ is whatever type we’re reducing a value to.
Historically I’ve found ‘Base’ here to be confusing, but here’s a neat trick to
help reason through it.
Remember that ‘Base’ is a type family, so for some appropriate ‘t’ and ‘a’,
‘Base t a’ is going to be a synonym for some other type. To figure out what
‘Base t a’ corresponds to for some concrete ‘t’ and ‘a’, we can ask GHCi via
this lesser-known command that evaluates type families:
> :kind! Base Nat Int
Base Nat Int :: *
= NatF Int
So in the ‘natsum’ example the algebra used with ‘cata’ must have type ‘NatF
Int -> Int’. This is pretty obvious for ‘cata’, but I initially found that
figuring out what type should be replaced for ‘Base’ exactly could be confusing
for some of the more exotic schemes.
As another example, we can use a catamorphism to implement ‘filter’ for our list type:
filterL :: (a -> Bool) -> List a -> List a
filterL p = cata alg where
alg NilF = nil
alg (ConsF x xs)
| p x = cons x xs
| otherwise = xs
It follows the same simple pattern: we define our semantics by interpreting
recursion-less constructors through an algebra, then pump it through ‘cata’.
Anamorphisms
These running examples are toys, but even here it’s really annoying to have to
type ‘succ (succ (succ (succ (succ (succ zero)))))’ to get a natural number
corresponding to six for debugging or what have you.
We can use an anamorphism to build a ‘Nat’ value from an ‘Int’:
nat :: Int -> Nat
nat = ana coalg where
coalg n
| n <= 0 = ZeroF
| otherwise = SuccF (n - 1)
Just as a small detail: to be descriptive, here I’ve used ‘coalg’ as the
argument to ‘ana’, for ‘coalgebra’.
Now the expression ‘nat 6’ will do the same for us as the more verbose example
above. As always, recursion is not part of the semantics; to have the integer
‘n’ we pass in correspond to the correct natural number, we use the successor
value of ‘n — 1’.
Paramorphisms
As an example, try to express a factorial on a natural number in terms of
‘cata’. It’s (apparently) doable, but an implementation is not immediately
clear.
A paramorphism will operate on an algebra that provides access to the input
argument corresponding to the running state of the recursion. Check out the
type of ‘para’ below:
para :: Foldable t => (Base t (t, a) -> a) -> t -> a
If we’re implementing a factorial on ‘Nat’ values then ‘t’ is going to
correspond to ‘Nat’ and ‘a’ is going to correspond to (say) ‘Integer’. Here it
might help to use the ‘:kind!’ trick to help reason through the requirements of
the algebra. We can ask GHCi to help us out:
> :kind! Base Nat (Nat, Int)
Base Nat (Nat, Int) :: *
= NatF (Nat, Int)
Side note: after doing this trick a few times you’ll probably find it much
easier to reason about type families sans-GHCi. In any case, we can now
implement an algebra corresponding to the required type:
natfac :: Nat -> Int
natfac = para alg where
alg ZeroF = 1
alg (SuccF (n, f)) = natsum (succ n) * f
Here there are some details to point out.
The type of our algebra is ‘NatF (Nat, Int) -> Int’; the value with the ‘Nat’
type, ‘n’, holds the most recent input argument used to compute the state of
the computation, ‘f’.
If you picture a factorial defined as
0! = 1
(k + 1)! = (k + 1) * k!
Then ‘n’ corresponds to ‘k’ and ‘f’ corresponds to ‘k!’. To compute the
factorial of the successor to ‘n’, we just convert ‘succ n’ to an integer (via
‘natsum’) and multiply it by ‘f’.
Paramorphisms tend to be pretty useful for a lot of mundane tasks. We can
easily implement ‘pred’ on natural numbers via ‘para’:
natpred :: Nat -> Nat
natpred = para alg where
alg ZeroF = zero
alg (SuccF (n, _)) = n
We can also implement ‘tail’ on lists. To check the type of the required
algebra we can again get some help from GHCi; here I’ll evaluate a general type
family, for illustration:
> :set -XRankNTypes
> :kind! forall a b. Base (List a) (List a, b)
forall a b. Base (List a) (List a, b) :: *
= forall a b. ListF a (Fix (ListF a), b)
Providing an algebra of the correct structure lets ‘tailL’ fall out as follows:
tailL :: List a -> List a
tailL = para alg where
alg NilF = nil
alg (ConsF _ (l, _)) = l
You can check that ‘tailL’ indeed returns the tail of its argument.
Hylomorphisms
Hylomorphisms can express general computation — corecursive production followed
by recursive consumption. Compared to the other type signatures in
recursion-schemes, ‘hylo’ is quite simple:
hylo :: Functor f => (f b -> b) -> (a -> f a) -> a -> b
It doesn’t even require the full structure built up for e.g. ‘cata’ and ‘ana’;
just very simple F-{co}algebras.
My favourite example hylomorphism is an absolutely beautiful implementation of
mergesort. I think it helps illustrate how recursion schemes can help tease out
incredibly simple structure in what could otherwise be a more involved problem.
Our input will be a Haskell list containing some orderable type. We’ll use it
to build a balanced binary tree via an anamorphism and then tear it down with a
catamorphism, merging lists together and sorting them as we go.
The resulting code looks like this:
mergeSort :: Ord a => [a] -> [a]
mergeSort = hylo alg coalg where
alg EmptyF = []
alg (LeafF c) = [c]
alg (NodeF l r) = merge l r
coalg [] = EmptyF
coalg [x] = LeafF x
coalg xs = NodeF l r where
(l, r) = splitAt (length xs `div` 2) xs
What’s more, the fusion achieved via this
technique is
really quite lovely.
Wrapping Up
Hopefully this article helps fuel any ‘programming via structured
recursion’ trend that might be ever-so-slowly growing.
When programming in a language like Haskell, a very natural pattern is to write
little embedded languages and mini-interpreters or compilers to accomplish
tasks. Typically these tiny embedded languages have a recursive structure, and
when you’re interpreting a recursive structure, you have use all these lovely
off-the-shelf strategies for recursion available to you to keep your programs
concise, modular, and efficient. The recursion-schemes library really has all
the built-in machinery you need to start using these things for real.
If you’re interested about hearing about using recursion schemes ‘for real’ I
recommend Tim Williams’s Exotic Tools For Exotic
Trades talk (for a motivating
example for the use of recursion schemes in production) or his talk on
recursion schemes from the London
Haskell User’s Group a few years ago.
So happy recursing! I’ve dropped the code from this article into a
gist.
Thanks to Maciej Woś for review and helpful comments.
06 Jul 2014
Automatic differentiation is one of those things that’s famous for not being as
famous as it should be (uh..). It’s useful, it’s convenient, and yet fewer
know about it than one would think.
This
article
(by one of the guys working on
Venture) is the single best
introduction you’ll probably find to AD, anywhere. It gives a wonderful
introduction to the basics, the subtleties, and the gotchas of the subject.
You should read it. I’m going to assume you have.
In particular, you should note this part:
[computing gradients] can be done — the method is called reverse mode — but
it introduces both code complexity and runtime cost in the form of managing
this storage (traditionally called the “tape”). In particular, the space
requirements of raw reverse mode are proportional to the runtime of f.
In some applications this can be somewhat inconvenient - picture iterative
gradient-based sampling algorithms like Hamiltonian Monte Carlo, its famous auto-tuning version
NUTS, and Riemannian manifold variants. Tom
noted in this reddit thread
that symbolic differentiation - which doesn’t need to deal with tapes and
infinitesimal-tracking - can often be orders of magnitude faster than AD for
this kind of problem. When running these algos we calculate gradients many,
many times, and the additional runtime cost of the reverse-mode AD dance can
add up.
An interesting question is whether or not this can this be mitigated
at all, and to what degree. In particular: can we use automatic
differentiation to implement efficient symbolic differentiation? Here’s a
possible attack plan:
- use an existing automatic differentiation implementation to calculate the
gradient for some target function at a point
- capture the symbolic expression for the gradient and optimize it by
eliminating common subexpressions or whatnot
- reify that expression as a function that we can evaluate for any input
- voila, (more) efficient gradient
Ed Kmett’s ‘ad’ library is the
best automatic differentiation library I know of, in terms of its balance
between power and accessibility. It can be used on arbitrary Haskell types
that are instances of the Num
typeclass and can carry out automatic
differentiation via a number of modes, so it’s very easy to get started with.
Rather than rolling my own AD implementation to try to do this sort of thing,
I’d much rather use his.
Start with a really basic language. In practice we’d be interested in
working with more expressive things, but this is sort of the minimal
interesting language capable of illustrating the problem:
import Numeric.AD
data Expr a =
Lit a
| Var String
| Add (Expr a) (Expr a)
| Sub (Expr a) (Expr a)
| Mul (Expr a) (Expr a)
deriving (Eq, Show)
instance Num a => Num (Expr a) where
fromInteger = Lit . fromInteger
e0 + e1 = Add e0 e1
e0 - e1 = Sub e0 e1
e0 * e1 = Mul e0 e1
We can already use Kmett’s ad library on these expressions to generate symbolic
expressions. We just have to write functions we’re interested in generically
(using +
, -
, and *
) and then call diff
or grad
or whatnot on them
with a concretely-typed argument. Some examples:
> :t diff (\x -> 2 * x ^ 2)
diff (\x -> 2 * x ^ 2) :: Num a => a -> a
> diff (\x -> 2 * x ^ 2) (Lit 1)
Mul (Add (Mul (Lit 1) (Lit 1)) (Mul (Lit 1) (Lit 1))) (Lit 2)
> grad (\[x, y] -> 2 * x ^ 2 + 3 * y) [Lit 1, Lit 2]
[ Add (Lit 0) (Add (Add (Lit 0) (Mul (Lit 1) (Add (Lit 0) (Mul (Lit 2) (Add
(Lit 0) (Mul (Lit 1) (Lit 1))))))) (Mul (Lit 1) (Add (Lit 0) (Mul (Lit 2) (Add
(Lit 0) (Mul (Lit 1) (Lit 1)))))))
, Add (Lit 0) (Add (Lit 0) (Mul (Lit 3) (Add (Lit 0) (Mul (Lit 1) (Lit 1)))))
]
It’s really easy to extract a proper derivative/gradient ‘function’ by
doing something like this:
> diff (\x -> 2 * x ^ 2) (Var "x")
Mul (Add (Mul (Var "x") (Lit 1)) (Mul (Lit 1) (Var "x"))) (Lit 2)
and then, given that expression, reifying a direct function for the derivative
by substituting over the captured variable and evaluating everything. Here’s
some initial machinery to handle that:
-- | Close an expression over some variable.
close :: Expr a -> String -> a -> Expr a
close (Add e0 e1) s x = Add (close e0 s x) (close e1 s x)
close (Sub e0 e1) s x = Sub (close e0 s x) (close e1 s x)
close (Mul e0 e1) s x = Mul (close e0 s x) (close e1 s x)
close (Var v) s x
| v == s = Lit x
| otherwise = Var v
close e _ _ = e
-- | Evaluate a closed expression.
eval :: Num a => Expr a -> a
eval (Lit d) = d
eval (Var _) = error "expression not closed"
eval (Add e0 e1) = eval e0 + eval e1
eval (Sub e0 e1) = eval e0 - eval e1
eval (Mul e0 e1) = eval e0 * eval e1
So, using this on the example above yields
> let testExpr = diff (\x -> 2 * x ^ 2) (Var "x")
> eval . close testExpr "x" $ 1
2
and it looks like a basic toDerivative
function for expressions could be
implemented as follows:
-- | Do some roundabout AD.
toDerivative expr = eval . close diffExpr "x" where
diffExpr = diff expr (Var "x")
But that’s a no go. ‘ad’ throws a type error, as presumably we’d be at risk of
perturbation confusion:
Couldn't match expected type ‘AD
s (Numeric.AD.Internal.Forward.Forward (Expr c))
-> AD s (Numeric.AD.Internal.Forward.Forward (Expr c))’
with actual type ‘t’
because type variable ‘s’ would escape its scope
This (rigid, skolem) type variable is bound by
a type expected by the context:
AD s (Numeric.AD.Internal.Forward.Forward (Expr c))
-> AD s (Numeric.AD.Internal.Forward.Forward (Expr c))
at ParamBasic.hs:174:14-32
Relevant bindings include
diffExpr :: Expr c (bound at ParamBasic.hs:174:3)
expr :: t (bound at ParamBasic.hs:173:14)
toDerivative :: t -> c -> c (bound at ParamBasic.hs:173:1)
In the first argument of ‘diff’, namely ‘expr’
In the expression: diff expr (Var "x")
Instead, we can use ad’s auto
combinator to write an alternate eval function:
autoEval :: Mode a => String -> Expr (Scalar a) -> a -> a
autoEval x expr = (`go` expr) where
go _ (Lit d) = auto d
go v (Var s)
| s == x = v
| otherwise = error "expression not closed"
go v (Add e0 e1) = go v e0 + go v e1
go v (Sub e0 e1) = go v e0 - go v e1
go v (Mul e0 e1) = go v e0 * go v e1
and using that, implement a working toDerivative
:
toDerivative :: Num a => String -> Expr (Expr a) -> Expr a
toDerivative v expr = diff (autoEval v expr)
which, though it has a weird-looking type, typechecks and does the trick:
> let d = toDerivative "x" (Mul (Lit 2) (Mul (Var "x") (Var "x"))) (Var "x")
Mul (Add (Mul (Var "x") (Lit 1)) (Mul (Lit 1) (Var "x"))) (Lit 2)
> eval . close "x" 1 $ d
4
So now we have access to a reified AST for a derivative (or gradient), which
can be tweaked and optimized as needed. Cool.
The available optimizations depend heavily on the underlying language. For
starters, there’s easy and universal stuff like this:
-- | Reduce superfluous expressions.
elimIdent :: (Num a, Eq a) => Expr a -> Expr a
elimIdent (Add (Lit 0) e) = elimIdent e
elimIdent (Add e (Lit 0)) = elimIdent e
elimIdent (Add e0 e1) = Add (elimIdent e0) (elimIdent e1)
elimIdent (Sub (Lit 0) e) = elimIdent e
elimIdent (Sub e (Lit 0)) = elimIdent e
elimIdent (Sub e0 e1) = Sub (elimIdent e0) (elimIdent e1)
elimIdent (Mul (Lit 1) e) = elimIdent e
elimIdent (Mul e (Lit 1)) = elimIdent e
elimIdent (Mul e0 e1) = Mul (elimIdent e0) (elimIdent e1)
elimIdent e = e
Which lets us do some work up-front:
> let e = 2 * Var "x" ^ 2 + Var "x" ^ 4
Add (Mul (Lit 2) (Mul (Var "x") (Var "x"))) (Mul (Mul (Var "x") (Var "x"))
(Mul (Var "x") (Var "x")))
> let ge = toDerivative "x" e
Add (Mul (Add (Mul (Var "x") (Lit 1)) (Mul (Lit 1) (Var "x"))) (Lit 2))
(Add (Mul (Mul (Var "x") (Var "x")) (Add (Mul (Var "x") (Lit 1)) (Mul
(Lit 1) (Var "x")))) (Mul (Add (Mul (Var "x") (Lit 1)) (Mul (Lit 1)
(Var "x"))) (Mul (Var "x") (Var "x"))))
> let geOptim = elimIdent ge
Add (Mul (Add (Var "x") (Var "x")) (Lit 2)) (Add (Mul (Mul (Var "x")
(Var "x")) (Add (Var "x") (Var "x"))) (Mul (Add (Var "x") (Var "x")) (Mul
(Var "x") (Var "x"))))
> eval . close "x" 1 $ geOptim
8
But there are also some more involved optimizations that can be useful for some
languages. The basic language I’ve been using above, for example, has no
explicit support for sharing common subexpressions. You’ll recall from one of my previous posts that we
have a variety of methods to do that in Haskell EDSLs, including some that
allow sharing to be observed without modifying the underlying language. We can
use data-reify
, for example, to observe any implicit sharing in expressions:
> reifyGraph $ geOptim
let [(1,AddF 2 6),(6,AddF 7 10),(10,MulF 11 12),(12,MulF 4 4),(11,AddF 4 4),
(7,MulF 8 9),(9,AddF 4 4),(8,MulF 4 4),(2,MulF 3 5),(5,LitF 2),(3,AddF 4 4),
(4,VarF "x")] in 1
And even make use of a handy library found on Hackage
for performing common subexpression elimination on graphs returned by
reifyGraph
:
> cse <$> reifyGraph geOptim
let [(5,LitF 2),(1,AddF 2 6),(3,AddF 4 4),(6,AddF 7 10),(2,MulF 3 5),
(10,MulF 3 8),(8,MulF 4 4),(7,MulF 8 3),(4,VarF "x")] in 1
With an appropriate graph evaluator
we can cut down the size of the syntax we have to traverse substantially.
Happy automasymbolic differentiating!